
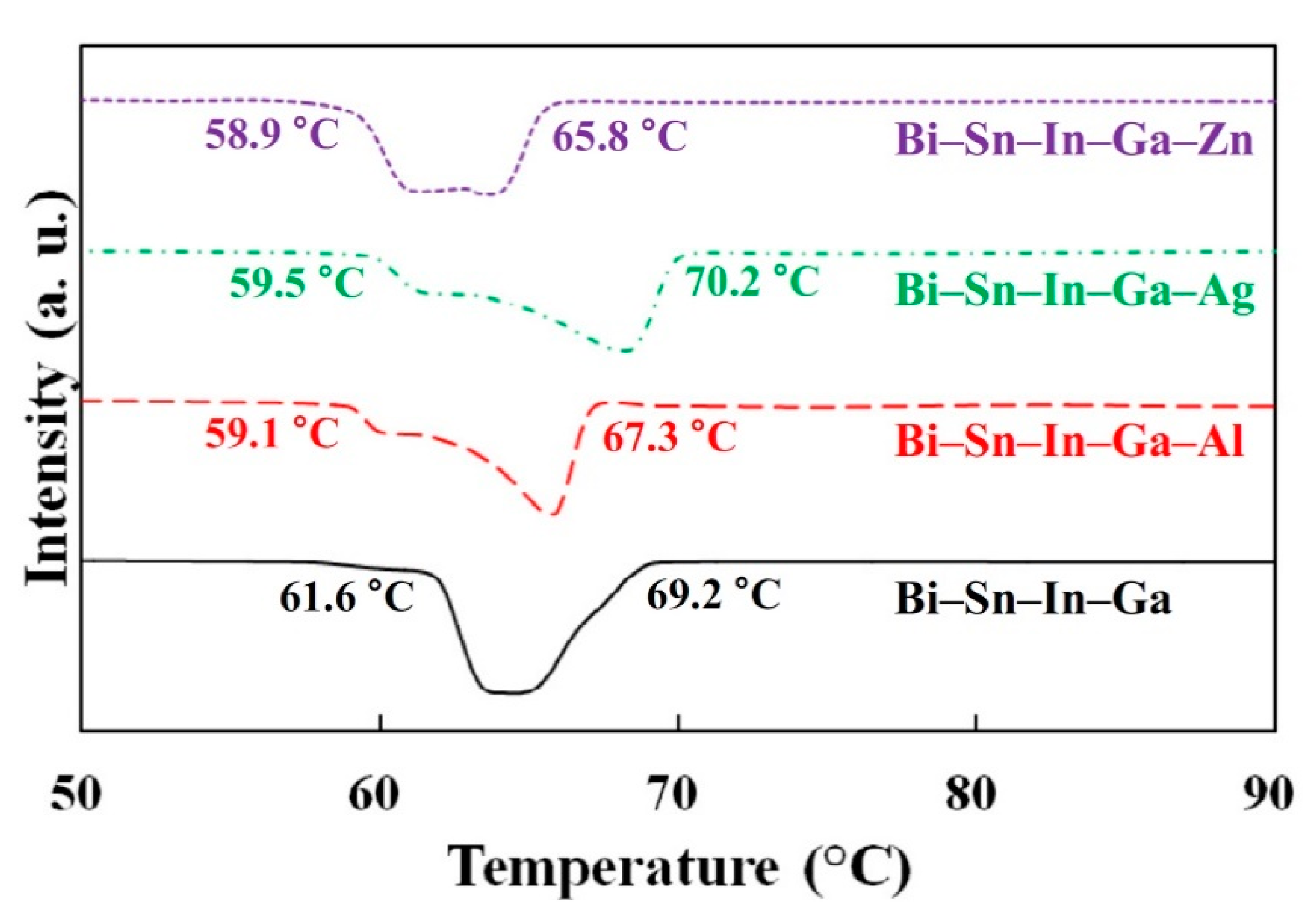
In this paper, we review the salient features of HEAs and summarize their core effects, phase structures, unconventional synthesis methods, and novel energy and environmental applications. Based on the exceptional synthesis methods, HEAs surprisingly afford numerous energy and environmental properties, which have endowed HEAs with promising applications. Therefore, HEAs have become an emerging class of advanced materials leading to a new field. The impressive mechanical properties, such as excellent strength, unforgettable corrosion resistance, and superior thermostability, are inherited and overwhelming compared with traditional alloys. one particle has all the energy in the universe and the rest have. When the way the energy is distributed changes from a less probable distribution (e.g.

which is a higher temperature, the increase of entropy A Paper read before. There is a constant amount of energy in the universe, but the way it is distributed is always changing. The principles are the same on a large scale but there is leakage of heat. Recently, high-entropy alloys (HEAs) have drawn enormous attention in diverse fields because of their distinctive concept and unique properties. Entropy is not energy entropy is how the energy in the universe is distributed. OctoHigh entropy alloys: Structural disorder and magnetic properties by Helmholtz Association of German Research Centres The Cantor alloy under study consists of chromium (gray). Accompanied by enhancements in the ability to fabricate materials for humans, alloy-based materials have advanced from binary alloy systems to complicated compositions along with affording newer applications, which can accelerate the evolution of civilization. Experiments show that DORL not only captures user interests well but also alleviates the Matthew effect.
#HIGH ENTROPY OFFLINE#
This leads to the main technical contribution of the work: Debiased model-based Offline RL (DORL) method.
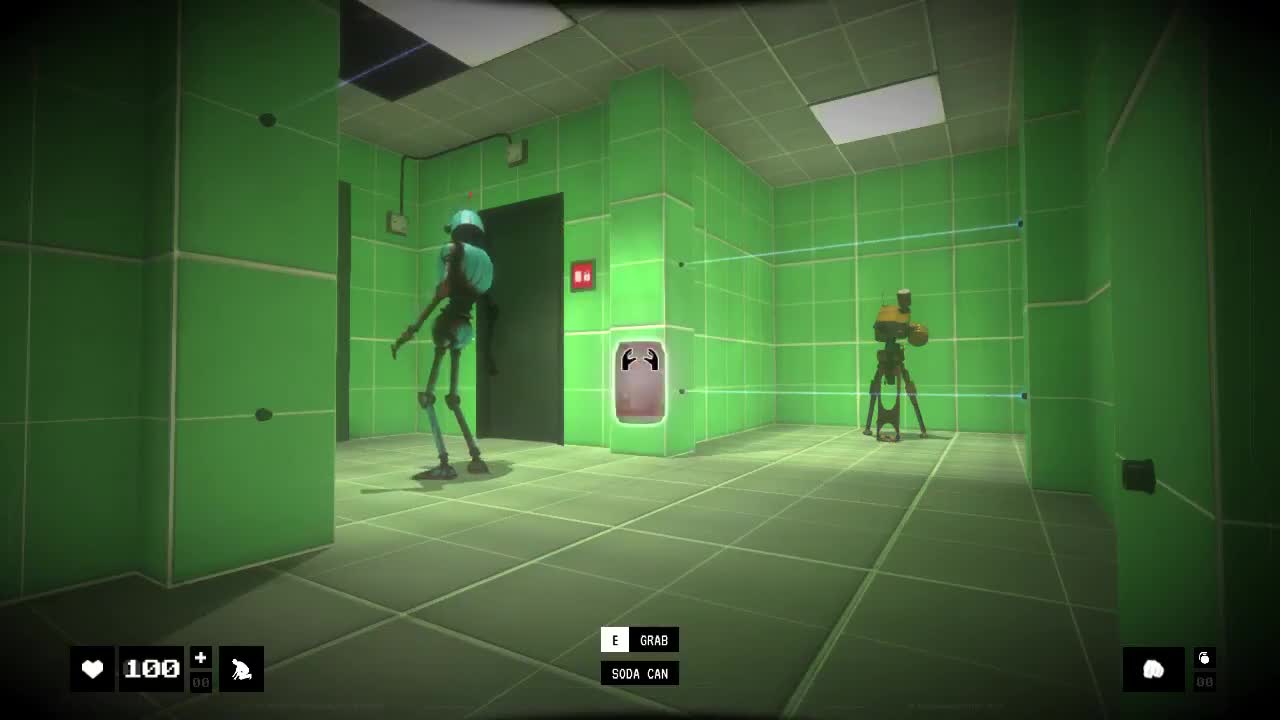
It inspires us to add a penalty term to relax the pessimism on states with high entropy of the logging policy and indirectly penalizes actions leading to less diverse states. Through theoretical analyses, we find that the conservatism of existing methods fails in pursuing users' long-term satisfaction. In this paper, we aim to alleviate the Matthew effect in offline RL-based recommendation. It is a notorious issue that needs to be addressed in practical recommender systems. But first things first, what is this information What ‘information’ am I referring to In simple words, we know that information is some facts learned about something or someone.
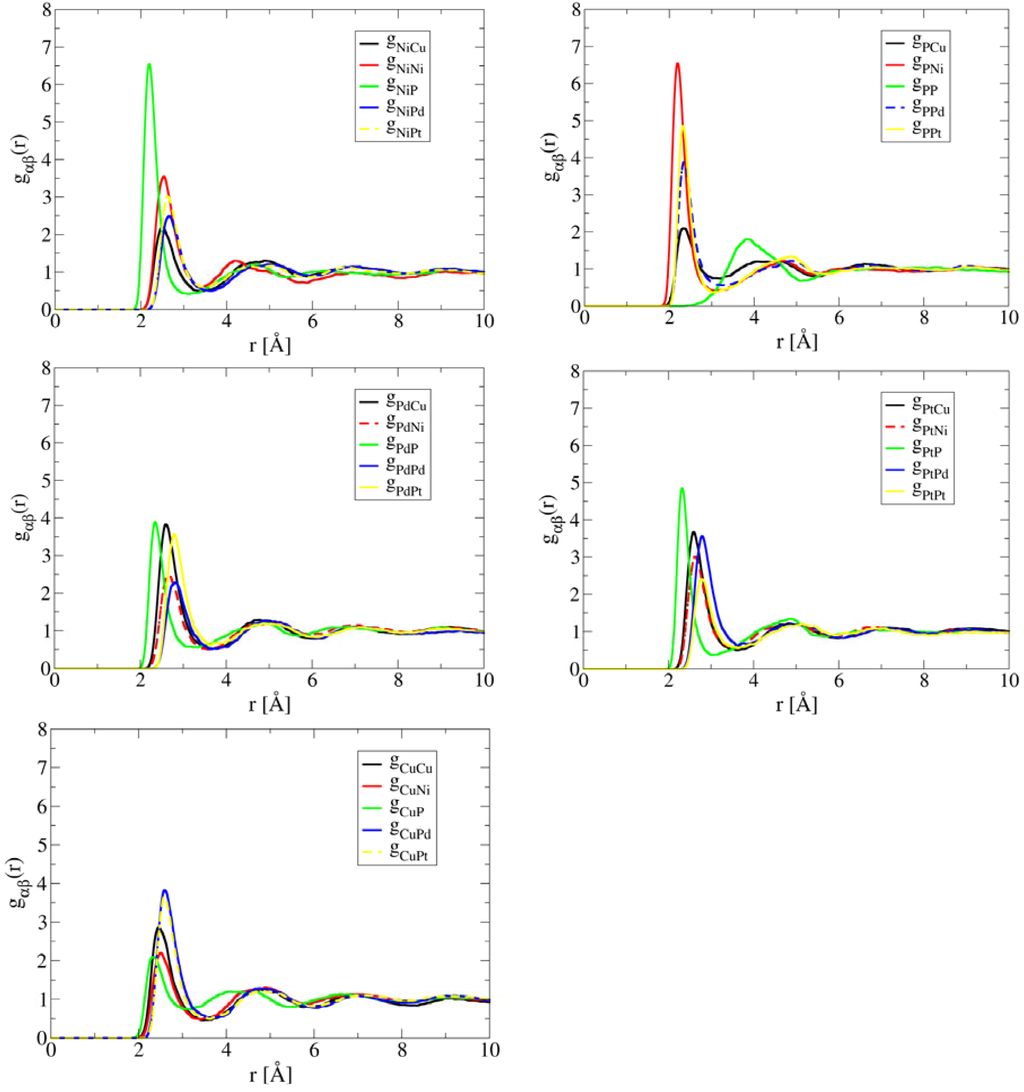
However, when applying such offline RL to recommendation, it will cause a severe Matthew effect, i.e., the rich get richer and the poor get poorer, by promoting popular items or categories while suppressing the less popular ones. The English meaning of the word entropy is: it is a state of disorder, confusion, and disorganization. To address it, existing methods employ conservatism, e.g., by constraining the learned policy to be close to behavior policies or punishing the rarely visited state-action pairs. Offline RL faces the value overestimation problem. Offline reinforcement learning (RL), a technology that offline learns a policy from logged data without the need to interact with online environments, has become a favorable choice in decision-making processes like interactive recommendation.
